Feature - Prediction Correlation
Collapse Tensorflow Coupon Figure
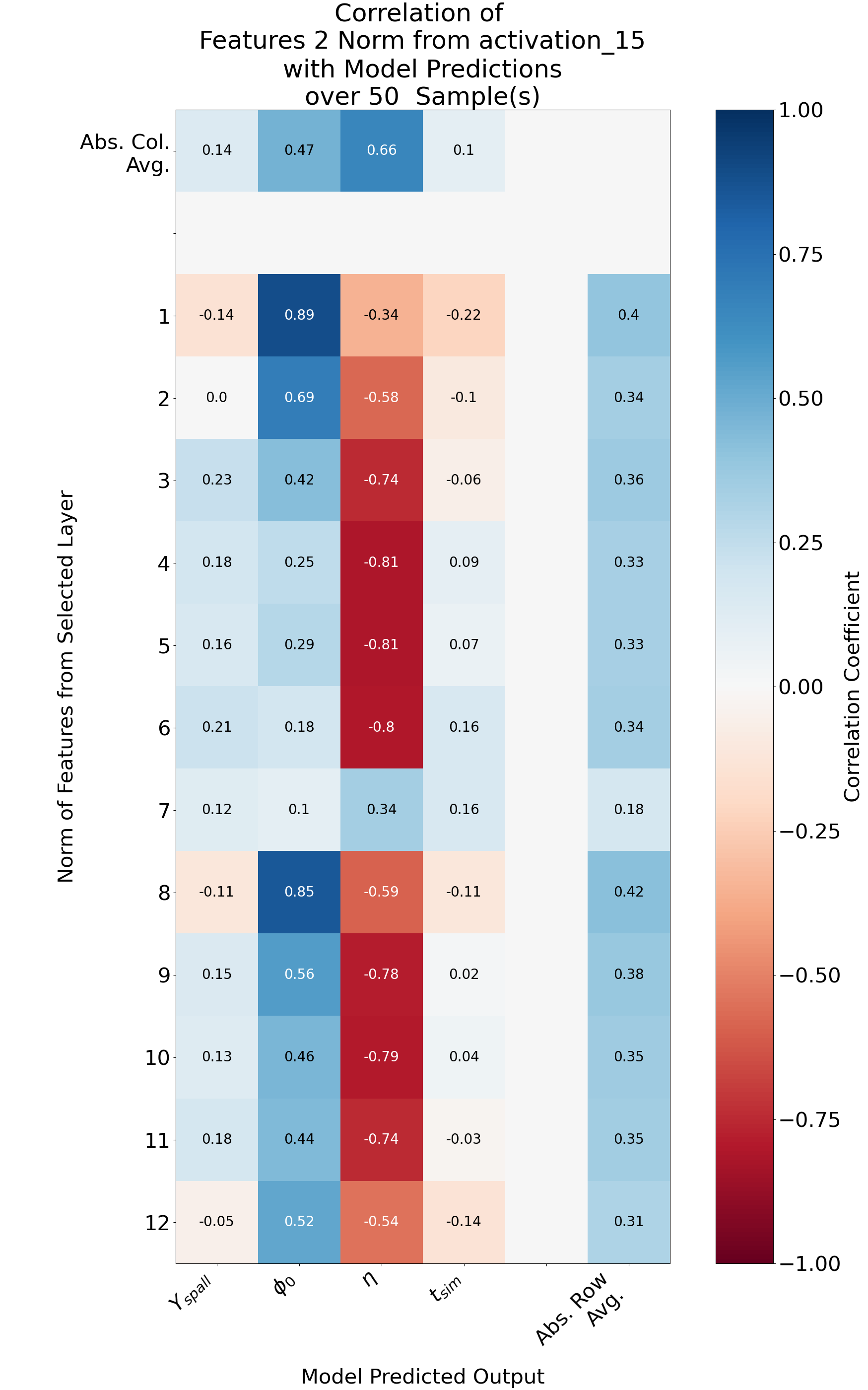
Correlation of \(L_2\) Norm of Coupon Features and Network Predictions
Collapse Pytorch Nested Cylinder Figure
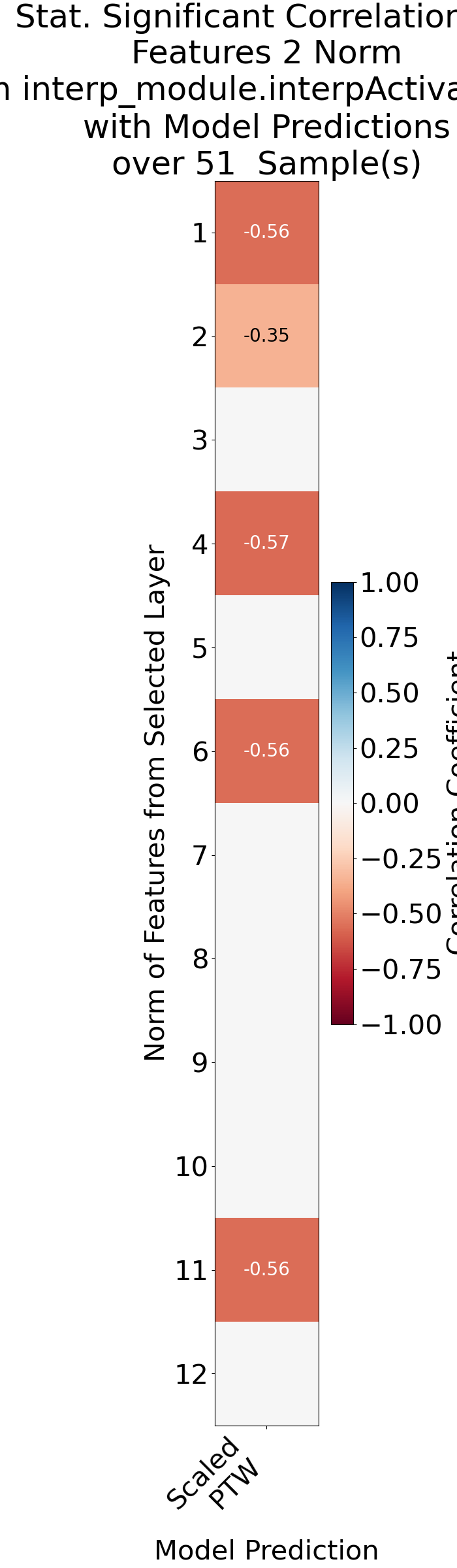
Statistically Significnat Correlations of L2 Norm of Nested Cylinder Features and Network Predictions
Code Documentation
- Generates a matrix of the cross-correlation-coefficient between the vector of feature norms across a given number of samples and the vector of model predictions across the same samples
Can plot all features (
-T All
) or some selected features (-T # #
)
- Calculates three correlation metrics:
2D cross-correlation
Partial correlation taking other features as confounding factors
Partial rank correlationtaking other features as confounding factors
- For each metric, generates a matrix for the:
Correlation coefficients
P-values
Statistically significnat correlation coeffieincts corresponding to a p-value less than some threshold
- Fixed key (
-XK
) specifies what subset of data to consider ‘None’ can be passed to consider any input with no restrictions
For coupon data, fixed keys must be in the form ‘tpl###’ or ‘idx#####’
For nested cylinder data, fixed keys must be in the form ‘id####’ or ‘idx#####’
Exports correlation coeffients as a pandas-readable .csv
-FL filepath
) OR-FL MAKE -NS #
)Input Line for TF Coupon Models:
python feature_pred_corr.py -P tensorflow -E coupon -M ../examples/tf_coupon/trained_pRad2TePla_model.h5 -IF pRad -ID ../examples/tf_coupon/data/ -DF ../examples/tf_coupon/coupon_design_file.csv -L activation_15 -T All -NR 2 -S ../examples/tf_coupon/figures/
Input Line for PYT Nested Cylinder Models:
python feature_pred_corr.py -P pytorch -E nestedcylinder -M ../examples/pyt_nestedcyl/trained_rho2PTW_model.pth -IF rho -ID ../examples/pyt_nestedcyl/data/ -DF ../examples/pyt_nestedcyl/nestedcyl_design_file.csv -L interp_module.interpActivations.10 -T All -NR 2 -S ../examples/pyt_nestedcyl/figures/
Arguments
Generates a matrix of the cross-correlation-coefficient between the vector of norms of features across a given number of samples and the vector of model predictionss across the same samples
usage: python feature_pred_corr.py [-h] [--PACKAGE] [--EXPERIMENT] [--MODEL]
[--INPUT_FIELD] [--INPUT_DIR] [--FILE_LIST]
[--DESIGN_FILE] [--PRINT_LAYERS]
[--PRINT_FEATURES] [--PRINT_FIELDS]
[--PRINT_KEYS] [--PRINT_SAMPLES] [--LAYER]
[--FEATURES [...]] [--SCLR_NORM]
[--FIXED_KEY] [--NUM_SAMPLES] [--SAVE_FIG]
Named Arguments
- --PACKAGE, -P
Possible choices: tensorflow, pytorch
Which python package was used to create the model
Default: “tensorflow”
- --EXPERIMENT, -E
Possible choices: coupon, nestedcylinder
Which experiment the model was trained on
Default: “coupon”
- --MODEL, -M
Model file
Default: “../examples/tf_coupon/trained_pRad2TePla_model.h5”
- --INPUT_FIELD, -IF
The radiographic/hydrodynamic field the model is trained on
Default: “pRad”
- --INPUT_DIR, -ID
Directory path where all of the .npz files are stored
Default: “../examples/tf_coupon/data/”
- --FILE_LIST, -FL
The .txt file containing a list of .npz file paths; use “MAKE” to generate a file list given an input directory (passed with -ID) and a number of samples (passed with -NS).
Default: “MAKE”
- --DESIGN_FILE, -DF
The .csv file with master design study parameters
Default: “../examples/tf_coupon/coupon_design_file.csv”
- --PRINT_LAYERS, -PL
Prints list of layer names in a model (passed with -M) and quits program
Default: False
- --PRINT_FEATURES, -PT
Prints number of features extracted by a layer (passed with -L) and quits program
Default: False
- --PRINT_FIELDS, -PF
Prints list of hydrodynamic/radiographic fields present in a given .npz file (passed with -IN) and quits program
Default: False
- --PRINT_KEYS, -PK
Prints list of choices for the fixed key avialable in a given input dirrectory (passed with -ID) and quits program
Default: False
- --PRINT_SAMPLES, -PS
Prints number of samples in a directory (passed with -ID) matching a fixed key (passed with -XK) and quits program
Default: False
- --LAYER, -L
Name of model layer that features will be extracted from
Default: “None”
- --FEATURES, -T
List of features to include; “Grid” plots all features in one figure using subplots; “All” plots all features each in a new figure; A list of integers can be passed to plot those features each in a new figure. Integer convention starts at 1.
Default: [‘All’]
- --SCLR_NORM, -NR
Possible choices: fro, nuc, inf, -inf, 0, 1, -1, 2, -2
How the extracted features will be normalized, resulting in a scalar value; for choices, see numpy.linalg.norm documentation.
Default: “2”
- --FIXED_KEY, -XK
The identifying string for some subset of all data samples; pass “None” to consider all samples
Default: “None”
- --NUM_SAMPLES, -NS
Number of samples to use; pass “All” to use all samples in a given input dirrectory (passed with -ID)
Default: “All”
- --SAVE_FIG, -S
Directory to save the outputs to.
Default: “../examples/tf_coupon/figures/”